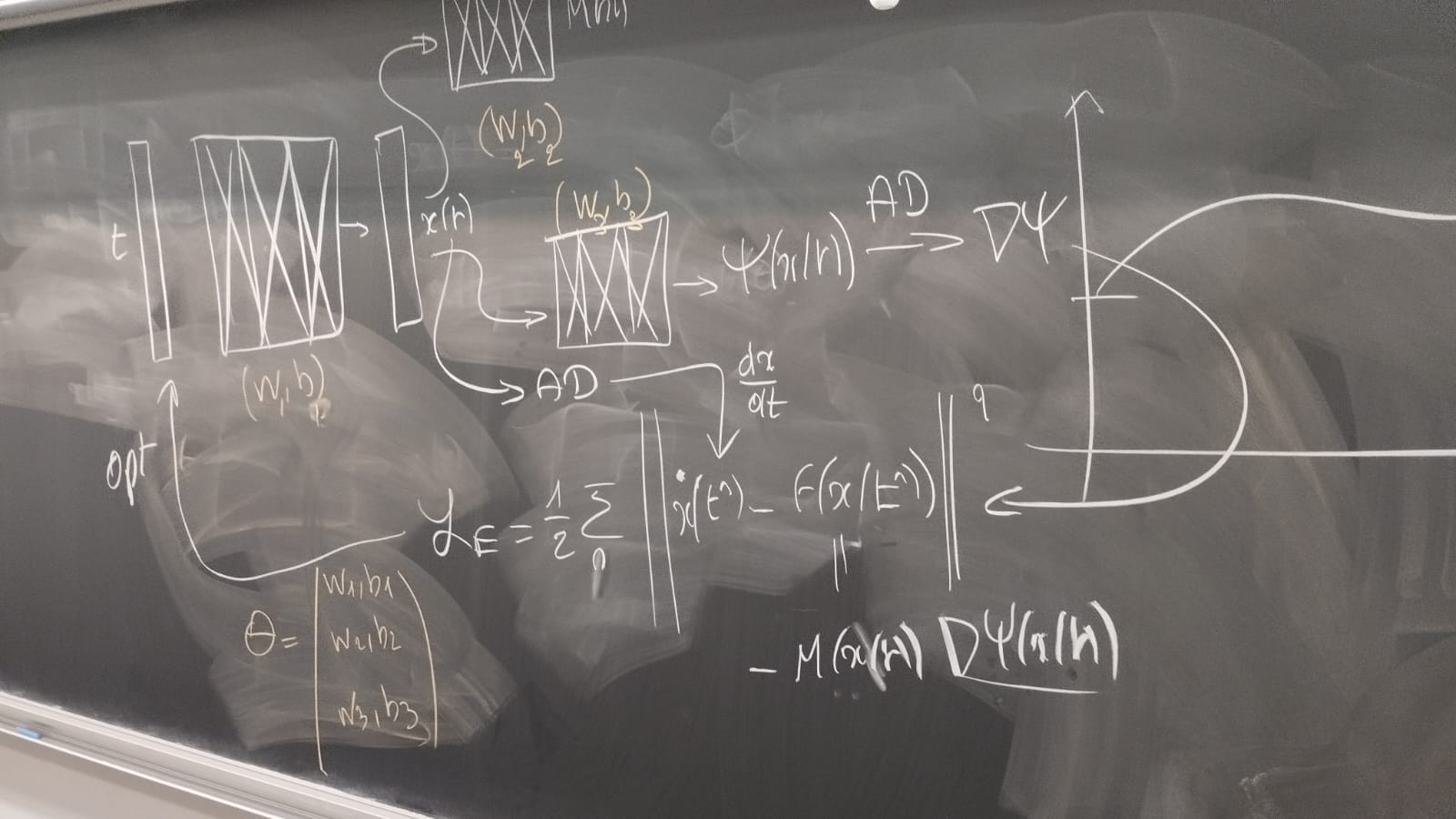
This course is a mathematical introduction to Scientific Machine Learning (SML). It introduces the theory of approximation: interpolation, linear and nonlinear regression, universal approximators, with a special focus on artificial neural networks (ANN). The concepts behind deep neural network will be explained. Another important topic of this course is the potential synergy between Mechanical modeling, Computer-assisted Engineering and Data-based Machine Learning approaches. Last but not least, this course is also intended to practically implement the methods and algorithms seen in the course. For that, modern development environments like Jupyter Notebooks with python as base programming language will be used.
- Introduction to artificial neural networks (ANN), shallow NN
- Introduction to numerical optimization. Application to nonlinear regression
- Dimensionality reduction : Singular Value Decomposition (SVD), reduced bases, greedy algorithms
- Computational acceleration of solutions of linear and nonlinear systems
- Dynamic Mode Decomposition (DMD) for dynamical systems
- Physics-aware modelling. Structure-preserving differential systems (Dissipative systems, symplectic system, Hamiltonian, GENERIC approach)
- Introduction to Physics-Informed Neural Networks (PINNs)
Presence to courses and computer works is mandatory. The course unit will be evaluated by the study of a Research article related to the course, with an oral presentation (A1-format poster).
- Responsable: De Vuyst Florian